The EN-9320TX-E is a 10 Gigabit Ethernet PCI Express Server Adapter designed for the high-speed PCI Express bus architecture. This meets the high-performance requirements such as large file transfers and HD video editing, which utilizes high-performance shared storage system to have improved server efficiency and network performance. Connecting the EN-9320TX-E with the RJ45 cable, your system instantly upgraded to superior performance in fast and reliable10Gbps high-speed. The EN-9320TX-E is an ideal selection to upgrade your server or workstation as the high-performance, low-power consumption and better-quality solution to complement the requirements of low latency, high bandwidth, high-throughput and cost-effective Gigabit Ethernet Adapter.

Advanced Features & Security The EN-9320TX-E features 802.1Q Virtual LAN (VLAN) tagging for Ethernet frames providing network management with a flexible and more secure environment. Moreover the card supports advanced features for best performance such as 16K Jumbo Frame and IEEE 802.3x Flow Control. Cost Effective and Easy Migration 10G Adapter The cost-effective solution of 10Gbps high-speed adapter on 10 Gigabit network connection – offering full compatibility to 10/5/2.5/1Gbps and 100Mbps networking standards and existing copper network. SPECIFICATIONS HARDWARE Attachment Interface PCI Express Rev.
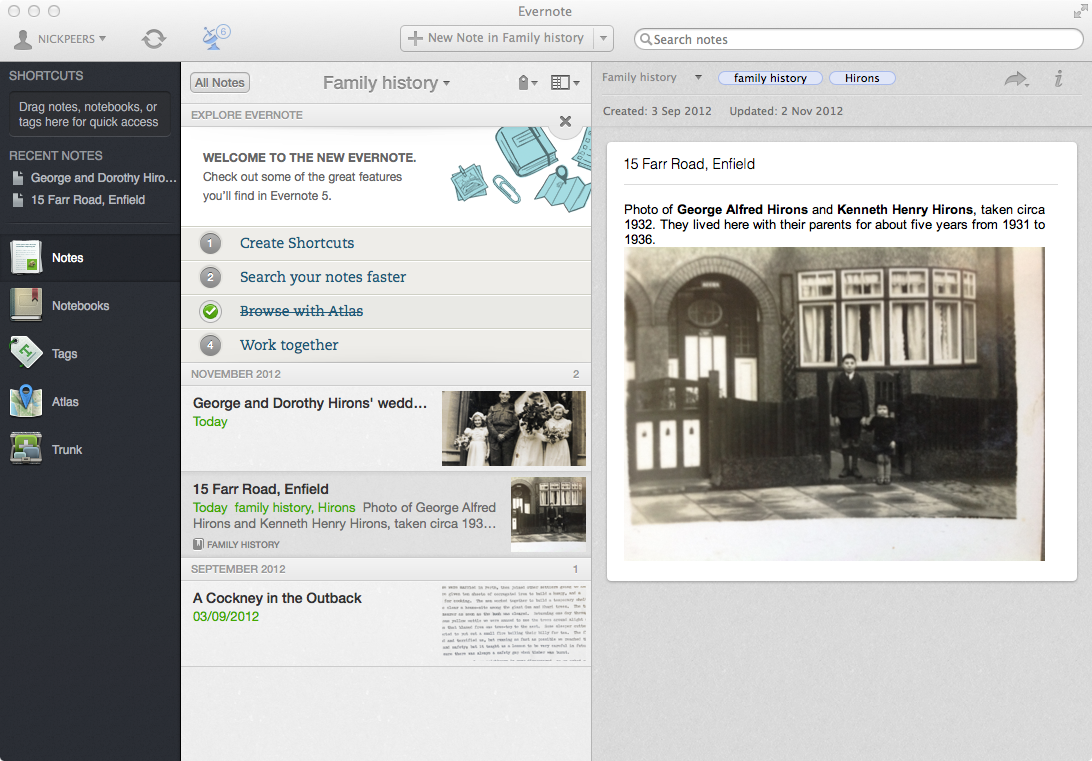
Evernote Mac 6.0.5: 100 Cpu Utilization Download
Libdispatch.dylib from Grand Central Dispatch is used internally by OSX's builtin implementation of BLAS called Accelerate when you do a numpy.dot calls. The GCD runtime does not work when programs call the POSIX fork syscall without using an exec syscall afterwards and therefore makes all Python programs that use the multiprocessing module prone to crash. Sklearn's GridsearchCV uses the Python multiprocessing module for parallelization.
Evernote Mac 6.0.5 100 Cpu Utilization
Under Python 3.4 and later you can force Python multiprocessing to use the instead of the default fork mode to workaround this problem, for instance at the beginning of the main file of your program: if name 'main': import multiprocessing as mp; mp.setstartmethod('forkserver') Alternatively, you can rebuild numpy from source and make it link against ATLAS or OpenBLAS instead of OSX Accelerate. The numpy developers are working on binary distributions that include either ATLAS or OpenBLAS by default.